Deep learning has many advantages, which make it a valuable tool for a variety of applications. However, it is not without its downsides. Deep learning’s value depends on context, goals, and resources. The following are some of the benefits of deep learning.
Advantages of Deep Learning
- Automated Feature Extraction: Deep learning excels in automatically identifying relevant data features. This reduces the need to manually engineer features, which is time-consuming and specific to a particular domain.
- Highly Accuracy: Deep learning models can be trained to achieve high accuracy for various tasks, such as speech processing and image recognition.
- Broad Applicability: Deep learning is versatile and can be used in a variety of domains from autonomous driving to financial analysis.
- Continuous Learning: Deep Learning models can be improved and updated over time using new data. This allows them to adapt to changes in patterns and trends of the data distribution.
- Hierarchical Data Representation: Deep networks are able to learn hierarchical patterns. They can capture both simple features in data and intricate relationships, leading them to more nuanced and richer insights.
- Real-time Processing: Certain deep-learning architectures optimized for parallel processing can achieve real time data analysis. This enables applications such as instant language translation or video analysis.
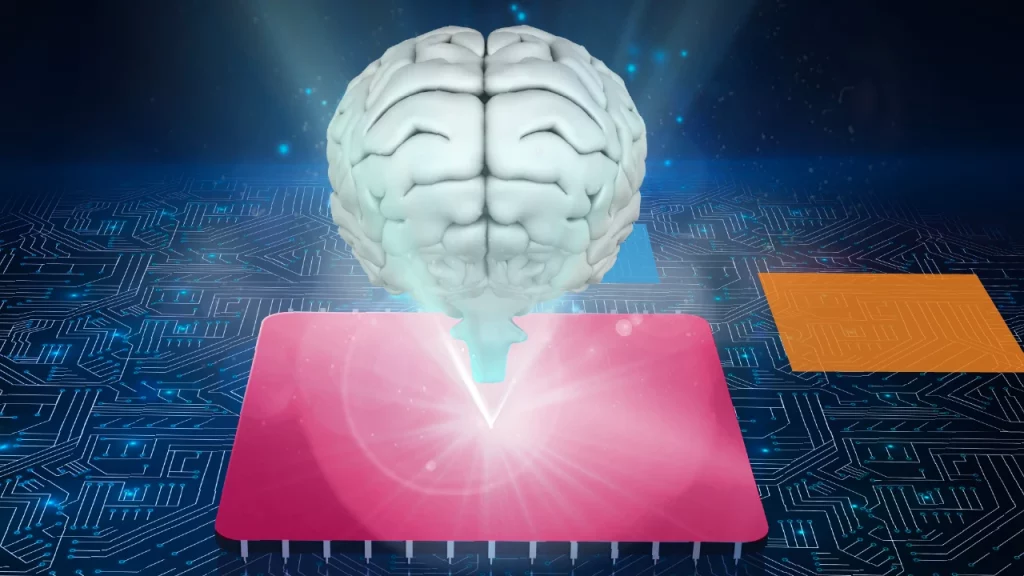
Disadvantages of Deep Learning
Deep learning has many benefits but also some downsides that raise public concern.
- Computational Intensity: Deep models require significant computing resources including powerful GPUs, and considerable time. This can lead to high energy costs and operational costs.
- Legal and Ethical Problems: Finding a balance between innovation and accountability while protecting individual and societal interest remains a major challenge for deep-learning technologies. AI is faced with issues such as data privacy, intellectual property rights and liability for harmful or biased outcomes. There are also some ethical issues, such as the possibility of reinforcing biases in the training data and lack of transparency of complex models.
- Interpretability Challenges: Deep learning models are complex and can be difficult to understand. Understanding why a deep learning model makes a certain decision can be difficult, affecting trust and adoption.
- Domain Specificity: Models that are trained to work in a particular domain may not be able to transfer well into other domains, requiring extensive retraining. This can take a lot of time and resources.